Faculty and Mentors
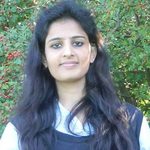
Prof. Raksha Sharma
CSE Dept., IIT Roorkee
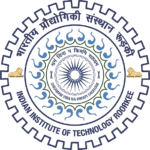
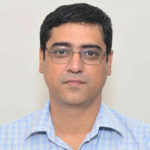
Prof. Sanjeev Manhas
ECE Dept., IIT Roorkee
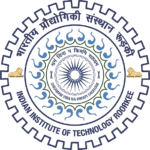
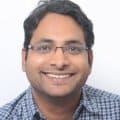
Mr. Sandeep Giri
Founder, CloudxLab
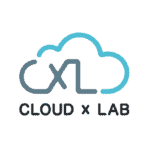
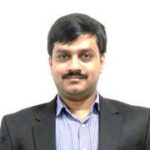
Mr. Abhinav Singh
Co-Founder, CloudxLab
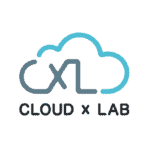
About the Course
This Machine Learning and AI Certification Program is a online course. This course covers some of the most trending and latest technologies in the market like Tensorflow 2.0, Generative Adversarial Networks (GANs) etc. The cutting edge content provided through this course will help you launch a career in the field of Machine Learning
Additionally, this course comes with our cloud lab access to gain the much needed hands-on experience to solve the real-world problems.
Upon successfully completing the course, you will get the certificate from E&ICT Academy, IIT Roorkee which you can use for progressing in your career and finding better opportunities.
Course Highlights
8 Months of Blended Learning
Cloud Lab Access
Work on about 25+ projects to get hands-on experience
Best In Class Curriculum
Timely Doubt Resolution
Certificate of Completion by E&ICT Academy, IIT Roorkee
Programming Languages and Tools
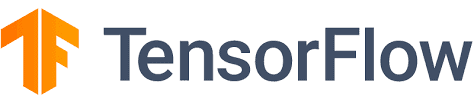
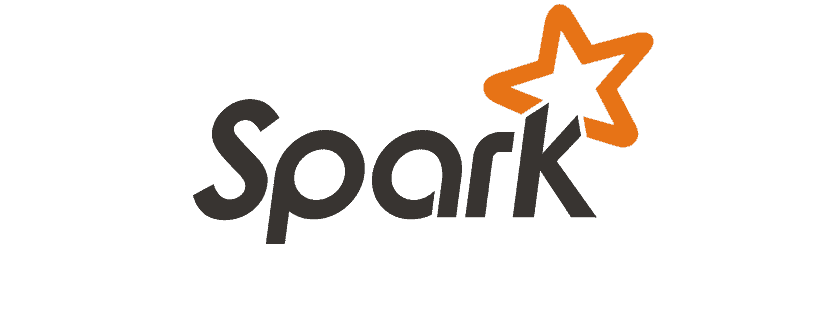
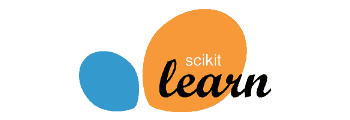
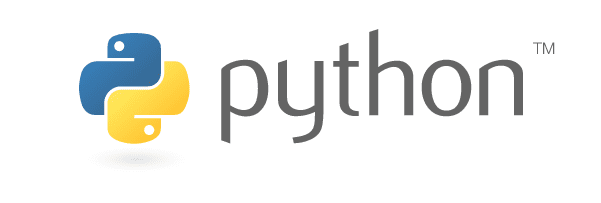
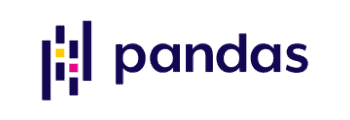
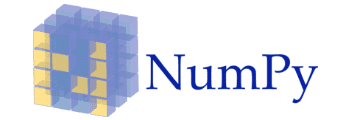
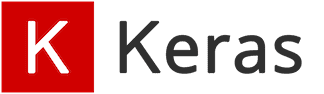
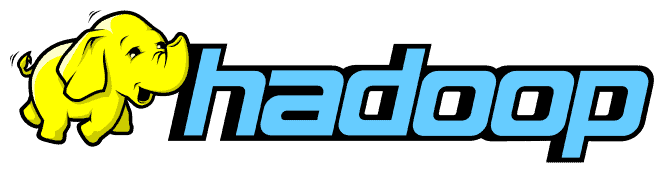
Sample Certificate
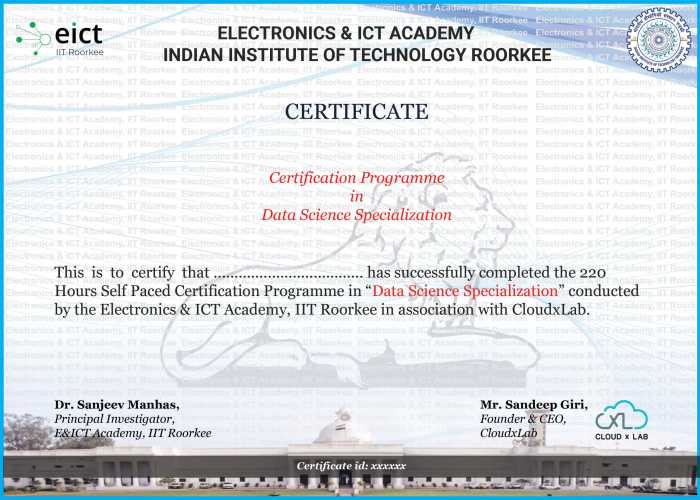
Curriculum
In this topic, we will cover concepts like different types of Machine Learning algorithms (Supervised, Unsupervised, Reinforcement) and challenges in Machine Learning. We will see examples of solving the problems using the traditional approach and why Machine Learning algorithms give far better accuracy than the traditional approach. This topic will give you a brief introduction to both Machine Learning and Deep Learning world.
We will start the course by learning concepts in Machine Learning. In this topic, we will build a machine learning model to predict housing pricing in California. By the end of this project, you will understand how to build machine learning pipelines to build a model. We will also cover concepts like data cleaning, preparing data for machine learning algorithms, exploring many different models, short-list the best one and fine-tuning the selected model
In this topic, we will train a model on the MNIST dataset to recognize handwritten digits. We will also learn various performance measures in classification like Confusion Matrix, Precision and Recall, and ROC Curve.
In this topic, we will learn various Machine Learning algorithms and concepts like Unsupervised Learning, Ensemble Learning, and Dimensionality Reduction
Reinforcement Learning is one of the most exciting fields of Machine Learning. Using Reinforcement Learning AlphaGo(system) defeated the world champion at the game of Go. Reinforcement Learning is an area of Machine Learning aimed at creating agents capable of taking actions in an environment in a way that maximizes rewards over time. In this topic, we will learn various concepts in Reinforcement Learning and experiment with OpenAI Gym.
Course on Big Data with Hadoop
We will start the course by learning concepts in Machine Learning. In this topic, we will build a machine learning model to predict housing pricing in California. By the end of this project, you will understand how to build machine learning pipelines to build a model. We will also cover concepts like data cleaning, preparing data for machine learning algorithms, exploring many different models, short-list the best one and fine-tuning the selected model